How to find sum of maximum K elements in range in array
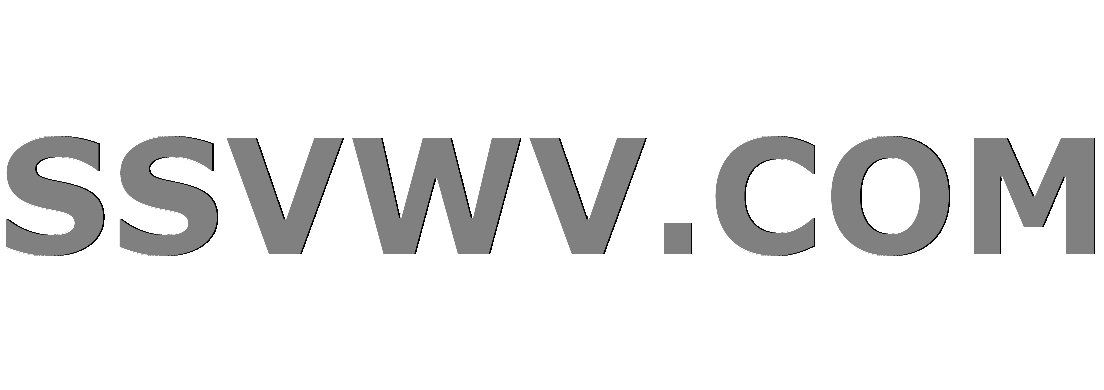
Multi tool use
.everyoneloves__top-leaderboard:empty,.everyoneloves__mid-leaderboard:empty,.everyoneloves__bot-mid-leaderboard:empty{ margin-bottom:0;
}
$begingroup$
Recently, I came up to the following problem:
Given array $A$ of size $n$ and integer $k$, We should answer $Q$ questions of the type:
for given range $[l, r]$ we should find sum of the $k$ maximum elements over all $A_i$ such that $l leq i leq r$.
Trivial solution would be to sort all values in the range and iterate over the k biggest elements, however this has complexity $O(QNlog N)$. After some searching on the internet, I found out that this can be solved by segment trees, however I couldn't think of proper use of segment trees for this problem.
Is it possible to solve this problem in complexity better than $O(QNlog N)$
data-structures search-trees
$endgroup$
add a comment |
$begingroup$
Recently, I came up to the following problem:
Given array $A$ of size $n$ and integer $k$, We should answer $Q$ questions of the type:
for given range $[l, r]$ we should find sum of the $k$ maximum elements over all $A_i$ such that $l leq i leq r$.
Trivial solution would be to sort all values in the range and iterate over the k biggest elements, however this has complexity $O(QNlog N)$. After some searching on the internet, I found out that this can be solved by segment trees, however I couldn't think of proper use of segment trees for this problem.
Is it possible to solve this problem in complexity better than $O(QNlog N)$
data-structures search-trees
$endgroup$
$begingroup$
There is eventually a trivial $O(Nk)$.
$endgroup$
– Vince
May 20 at 8:07
$begingroup$
Taking $Q = 1$, you are effectively not allowing any preprocessing at all.
$endgroup$
– Yuval Filmus
May 20 at 9:52
add a comment |
$begingroup$
Recently, I came up to the following problem:
Given array $A$ of size $n$ and integer $k$, We should answer $Q$ questions of the type:
for given range $[l, r]$ we should find sum of the $k$ maximum elements over all $A_i$ such that $l leq i leq r$.
Trivial solution would be to sort all values in the range and iterate over the k biggest elements, however this has complexity $O(QNlog N)$. After some searching on the internet, I found out that this can be solved by segment trees, however I couldn't think of proper use of segment trees for this problem.
Is it possible to solve this problem in complexity better than $O(QNlog N)$
data-structures search-trees
$endgroup$
Recently, I came up to the following problem:
Given array $A$ of size $n$ and integer $k$, We should answer $Q$ questions of the type:
for given range $[l, r]$ we should find sum of the $k$ maximum elements over all $A_i$ such that $l leq i leq r$.
Trivial solution would be to sort all values in the range and iterate over the k biggest elements, however this has complexity $O(QNlog N)$. After some searching on the internet, I found out that this can be solved by segment trees, however I couldn't think of proper use of segment trees for this problem.
Is it possible to solve this problem in complexity better than $O(QNlog N)$
data-structures search-trees
data-structures search-trees
edited May 20 at 9:25
someone12321
asked May 20 at 7:40
someone12321someone12321
6853 silver badges13 bronze badges
6853 silver badges13 bronze badges
$begingroup$
There is eventually a trivial $O(Nk)$.
$endgroup$
– Vince
May 20 at 8:07
$begingroup$
Taking $Q = 1$, you are effectively not allowing any preprocessing at all.
$endgroup$
– Yuval Filmus
May 20 at 9:52
add a comment |
$begingroup$
There is eventually a trivial $O(Nk)$.
$endgroup$
– Vince
May 20 at 8:07
$begingroup$
Taking $Q = 1$, you are effectively not allowing any preprocessing at all.
$endgroup$
– Yuval Filmus
May 20 at 9:52
$begingroup$
There is eventually a trivial $O(Nk)$.
$endgroup$
– Vince
May 20 at 8:07
$begingroup$
There is eventually a trivial $O(Nk)$.
$endgroup$
– Vince
May 20 at 8:07
$begingroup$
Taking $Q = 1$, you are effectively not allowing any preprocessing at all.
$endgroup$
– Yuval Filmus
May 20 at 9:52
$begingroup$
Taking $Q = 1$, you are effectively not allowing any preprocessing at all.
$endgroup$
– Yuval Filmus
May 20 at 9:52
add a comment |
2 Answers
2
active
oldest
votes
$begingroup$
This answer refers to the version of the question in which the interval $[l,r]$ refers to the values of the elements rather than their indices.
Without preprocessing: You can extract all elements in the range $[l,r]$ in linear time. You can then use the linear time selection algorithm to find the $k$'th largest element, and then you can easily find the sum of the $k$ maximum elements. Overall, you can answer each query in $O(n)$.
With preprocessing: Sort the array, and compute running sums. Given $l,r$, locate the subset of the array corresponding to this range using binary search, and then use the running sums to compute the sum of the $k$ largest elements in $O(1)$. Overall, this uses $O(nlog n)$ preprocessing and $O(log n)$ time per query.
$endgroup$
$begingroup$
@someone12321: do you mean O(n) is too slow, or that the constant factors are too high in any real implementation of it on normal CPUs? (too many passes over huge data costing a lot of memory bandwidth?)
$endgroup$
– Peter Cordes
May 20 at 9:59
$begingroup$
@PeterCordes For this version of the problem in the above answer, there is O(logN) solution per query.
$endgroup$
– someone12321
May 20 at 10:14
add a comment |
$begingroup$
This answer refers to the version of the question in which the range $[l,r]$ refers to indices of the array, and in which we have $Q$ queries. The question asked whether we could beat $O(Qnlog n)$.
Without preprocessing: Using the linear time selection algorithm, you can answer each query in $O(n)$, for a total of $O(Qn)$, which is better than $O(Qnlog n)$.
With preprocessing: Assume for simplicity that $n$ is a power of 2. We consider $log n$ layers, the first consisting of $n$ intervals of length 1, the second of $n/2$ intervals of length 2, and so on: the $k$th consists of $n/2^k$ intervals of length $k$. We sort all of the intervals in $O(nlog n)$ by "merging up the tree". At query time, we partition the input interval into $O(log n)$ stored intervals, and them return the answer in $O(klog n)$ by merging the $k$th largest elements from each.
We can improve the query time as follows. Along with the layered intervals, we also compute their running sums. Let us consider the $O(log n)$ layered intervals $I_1,ldots,I_m$ whose union is the given interval $[l,r]$. The $k$ largest elements of $[l,r]$ are composed of the $k_1,ldots,k_m$ largest elements in $I_1,ldots,I_m$. If we could determine $k_1,ldots,k_m$, then we could compute the answer in $O(m) = O(log n)$ using the running sums.
We can determine $k_1,ldots,k_m$ as follows. For each element $x in I_1$, we can count how many elements are larger than $x$ in time $O(mlog k) = O(log n log k)$ using binary search. By doing binary search on the top $k$ elements of $I_1$, we can determine $k_1$ in time $O(log n log^2 k)$. Continuing in this way, we can determine $k_1,ldots,k_m$ in time $O(log^2 n log^2 k)$.
This solution has preprocessing time $O(nlog n)$ and query time $O(log^2 n log^2 k)$.
We can obtain other tradeoffs by varying the fan-in of the tree. Suppose that the fan-in is $n^{1/d}$. For each internal node in the tree, we sort each interval-of-intervals within its children. At level $i$ we have $n^{i/d}$ vertices, each has $n^{2/d}$ intervals-of-intervals, each of length at most $n^{1-i/d}$, so sorting all intervals-of-intervals at level $i$ takes time $n^{1+2/d}$, for a total preprocessing time of $O(dn^{1+2/d})$. In query time, we can break $[l,r]$ into a union of $O(d)$ intervals, for a total query time of $O(d^2log^2 k)$.
Stated differently, for each $epsilon > 0$ this gives an algorithm with preprocessing time $O(epsilon^{-1} n^{1+epsilon})$ and query time $O(epsilon^{-2} log^2 k)$.
$endgroup$
$begingroup$
I don't think that this improves the things a lot, since k can be up to n.
$endgroup$
– someone12321
May 20 at 10:16
$begingroup$
You can precompute all the answers in $O(n^3)$, and then the time per query is $O(1)$.
$endgroup$
– Yuval Filmus
May 20 at 10:22
$begingroup$
We can even precompute all the answer in $O(n^2 log n)$ however this is very slow and memory consuming solution.
$endgroup$
– someone12321
May 20 at 10:25
$begingroup$
How about query time $O(log^4 n)$?
$endgroup$
– Yuval Filmus
May 20 at 10:45
$begingroup$
Recently, I read about persistent segment tree, and I think that we can solve this with persistent segment tree, for each prefix of the array we store one segment tree whose leafs actually represent the count of some number $x$. Then to find the sum we actually do binary search on two prefixes of all the roots we save, however I'm not really sure how to finish this and implement since we can have bigger numbers and even duplicates. Careful implementations can run in $O(log N)$
$endgroup$
– someone12321
May 20 at 13:12
add a comment |
Your Answer
StackExchange.ready(function() {
var channelOptions = {
tags: "".split(" "),
id: "419"
};
initTagRenderer("".split(" "), "".split(" "), channelOptions);
StackExchange.using("externalEditor", function() {
// Have to fire editor after snippets, if snippets enabled
if (StackExchange.settings.snippets.snippetsEnabled) {
StackExchange.using("snippets", function() {
createEditor();
});
}
else {
createEditor();
}
});
function createEditor() {
StackExchange.prepareEditor({
heartbeatType: 'answer',
autoActivateHeartbeat: false,
convertImagesToLinks: false,
noModals: true,
showLowRepImageUploadWarning: true,
reputationToPostImages: null,
bindNavPrevention: true,
postfix: "",
imageUploader: {
brandingHtml: "Powered by u003ca class="icon-imgur-white" href="https://imgur.com/"u003eu003c/au003e",
contentPolicyHtml: "User contributions licensed under u003ca href="https://creativecommons.org/licenses/by-sa/3.0/"u003ecc by-sa 3.0 with attribution requiredu003c/au003e u003ca href="https://stackoverflow.com/legal/content-policy"u003e(content policy)u003c/au003e",
allowUrls: true
},
onDemand: true,
discardSelector: ".discard-answer"
,immediatelyShowMarkdownHelp:true
});
}
});
Sign up or log in
StackExchange.ready(function () {
StackExchange.helpers.onClickDraftSave('#login-link');
});
Sign up using Google
Sign up using Facebook
Sign up using Email and Password
Post as a guest
Required, but never shown
StackExchange.ready(
function () {
StackExchange.openid.initPostLogin('.new-post-login', 'https%3a%2f%2fcs.stackexchange.com%2fquestions%2f109579%2fhow-to-find-sum-of-maximum-k-elements-in-range-in-array%23new-answer', 'question_page');
}
);
Post as a guest
Required, but never shown
2 Answers
2
active
oldest
votes
2 Answers
2
active
oldest
votes
active
oldest
votes
active
oldest
votes
$begingroup$
This answer refers to the version of the question in which the interval $[l,r]$ refers to the values of the elements rather than their indices.
Without preprocessing: You can extract all elements in the range $[l,r]$ in linear time. You can then use the linear time selection algorithm to find the $k$'th largest element, and then you can easily find the sum of the $k$ maximum elements. Overall, you can answer each query in $O(n)$.
With preprocessing: Sort the array, and compute running sums. Given $l,r$, locate the subset of the array corresponding to this range using binary search, and then use the running sums to compute the sum of the $k$ largest elements in $O(1)$. Overall, this uses $O(nlog n)$ preprocessing and $O(log n)$ time per query.
$endgroup$
$begingroup$
@someone12321: do you mean O(n) is too slow, or that the constant factors are too high in any real implementation of it on normal CPUs? (too many passes over huge data costing a lot of memory bandwidth?)
$endgroup$
– Peter Cordes
May 20 at 9:59
$begingroup$
@PeterCordes For this version of the problem in the above answer, there is O(logN) solution per query.
$endgroup$
– someone12321
May 20 at 10:14
add a comment |
$begingroup$
This answer refers to the version of the question in which the interval $[l,r]$ refers to the values of the elements rather than their indices.
Without preprocessing: You can extract all elements in the range $[l,r]$ in linear time. You can then use the linear time selection algorithm to find the $k$'th largest element, and then you can easily find the sum of the $k$ maximum elements. Overall, you can answer each query in $O(n)$.
With preprocessing: Sort the array, and compute running sums. Given $l,r$, locate the subset of the array corresponding to this range using binary search, and then use the running sums to compute the sum of the $k$ largest elements in $O(1)$. Overall, this uses $O(nlog n)$ preprocessing and $O(log n)$ time per query.
$endgroup$
$begingroup$
@someone12321: do you mean O(n) is too slow, or that the constant factors are too high in any real implementation of it on normal CPUs? (too many passes over huge data costing a lot of memory bandwidth?)
$endgroup$
– Peter Cordes
May 20 at 9:59
$begingroup$
@PeterCordes For this version of the problem in the above answer, there is O(logN) solution per query.
$endgroup$
– someone12321
May 20 at 10:14
add a comment |
$begingroup$
This answer refers to the version of the question in which the interval $[l,r]$ refers to the values of the elements rather than their indices.
Without preprocessing: You can extract all elements in the range $[l,r]$ in linear time. You can then use the linear time selection algorithm to find the $k$'th largest element, and then you can easily find the sum of the $k$ maximum elements. Overall, you can answer each query in $O(n)$.
With preprocessing: Sort the array, and compute running sums. Given $l,r$, locate the subset of the array corresponding to this range using binary search, and then use the running sums to compute the sum of the $k$ largest elements in $O(1)$. Overall, this uses $O(nlog n)$ preprocessing and $O(log n)$ time per query.
$endgroup$
This answer refers to the version of the question in which the interval $[l,r]$ refers to the values of the elements rather than their indices.
Without preprocessing: You can extract all elements in the range $[l,r]$ in linear time. You can then use the linear time selection algorithm to find the $k$'th largest element, and then you can easily find the sum of the $k$ maximum elements. Overall, you can answer each query in $O(n)$.
With preprocessing: Sort the array, and compute running sums. Given $l,r$, locate the subset of the array corresponding to this range using binary search, and then use the running sums to compute the sum of the $k$ largest elements in $O(1)$. Overall, this uses $O(nlog n)$ preprocessing and $O(log n)$ time per query.
edited May 20 at 9:50
answered May 20 at 8:32


Yuval FilmusYuval Filmus
205k15 gold badges200 silver badges365 bronze badges
205k15 gold badges200 silver badges365 bronze badges
$begingroup$
@someone12321: do you mean O(n) is too slow, or that the constant factors are too high in any real implementation of it on normal CPUs? (too many passes over huge data costing a lot of memory bandwidth?)
$endgroup$
– Peter Cordes
May 20 at 9:59
$begingroup$
@PeterCordes For this version of the problem in the above answer, there is O(logN) solution per query.
$endgroup$
– someone12321
May 20 at 10:14
add a comment |
$begingroup$
@someone12321: do you mean O(n) is too slow, or that the constant factors are too high in any real implementation of it on normal CPUs? (too many passes over huge data costing a lot of memory bandwidth?)
$endgroup$
– Peter Cordes
May 20 at 9:59
$begingroup$
@PeterCordes For this version of the problem in the above answer, there is O(logN) solution per query.
$endgroup$
– someone12321
May 20 at 10:14
$begingroup$
@someone12321: do you mean O(n) is too slow, or that the constant factors are too high in any real implementation of it on normal CPUs? (too many passes over huge data costing a lot of memory bandwidth?)
$endgroup$
– Peter Cordes
May 20 at 9:59
$begingroup$
@someone12321: do you mean O(n) is too slow, or that the constant factors are too high in any real implementation of it on normal CPUs? (too many passes over huge data costing a lot of memory bandwidth?)
$endgroup$
– Peter Cordes
May 20 at 9:59
$begingroup$
@PeterCordes For this version of the problem in the above answer, there is O(logN) solution per query.
$endgroup$
– someone12321
May 20 at 10:14
$begingroup$
@PeterCordes For this version of the problem in the above answer, there is O(logN) solution per query.
$endgroup$
– someone12321
May 20 at 10:14
add a comment |
$begingroup$
This answer refers to the version of the question in which the range $[l,r]$ refers to indices of the array, and in which we have $Q$ queries. The question asked whether we could beat $O(Qnlog n)$.
Without preprocessing: Using the linear time selection algorithm, you can answer each query in $O(n)$, for a total of $O(Qn)$, which is better than $O(Qnlog n)$.
With preprocessing: Assume for simplicity that $n$ is a power of 2. We consider $log n$ layers, the first consisting of $n$ intervals of length 1, the second of $n/2$ intervals of length 2, and so on: the $k$th consists of $n/2^k$ intervals of length $k$. We sort all of the intervals in $O(nlog n)$ by "merging up the tree". At query time, we partition the input interval into $O(log n)$ stored intervals, and them return the answer in $O(klog n)$ by merging the $k$th largest elements from each.
We can improve the query time as follows. Along with the layered intervals, we also compute their running sums. Let us consider the $O(log n)$ layered intervals $I_1,ldots,I_m$ whose union is the given interval $[l,r]$. The $k$ largest elements of $[l,r]$ are composed of the $k_1,ldots,k_m$ largest elements in $I_1,ldots,I_m$. If we could determine $k_1,ldots,k_m$, then we could compute the answer in $O(m) = O(log n)$ using the running sums.
We can determine $k_1,ldots,k_m$ as follows. For each element $x in I_1$, we can count how many elements are larger than $x$ in time $O(mlog k) = O(log n log k)$ using binary search. By doing binary search on the top $k$ elements of $I_1$, we can determine $k_1$ in time $O(log n log^2 k)$. Continuing in this way, we can determine $k_1,ldots,k_m$ in time $O(log^2 n log^2 k)$.
This solution has preprocessing time $O(nlog n)$ and query time $O(log^2 n log^2 k)$.
We can obtain other tradeoffs by varying the fan-in of the tree. Suppose that the fan-in is $n^{1/d}$. For each internal node in the tree, we sort each interval-of-intervals within its children. At level $i$ we have $n^{i/d}$ vertices, each has $n^{2/d}$ intervals-of-intervals, each of length at most $n^{1-i/d}$, so sorting all intervals-of-intervals at level $i$ takes time $n^{1+2/d}$, for a total preprocessing time of $O(dn^{1+2/d})$. In query time, we can break $[l,r]$ into a union of $O(d)$ intervals, for a total query time of $O(d^2log^2 k)$.
Stated differently, for each $epsilon > 0$ this gives an algorithm with preprocessing time $O(epsilon^{-1} n^{1+epsilon})$ and query time $O(epsilon^{-2} log^2 k)$.
$endgroup$
$begingroup$
I don't think that this improves the things a lot, since k can be up to n.
$endgroup$
– someone12321
May 20 at 10:16
$begingroup$
You can precompute all the answers in $O(n^3)$, and then the time per query is $O(1)$.
$endgroup$
– Yuval Filmus
May 20 at 10:22
$begingroup$
We can even precompute all the answer in $O(n^2 log n)$ however this is very slow and memory consuming solution.
$endgroup$
– someone12321
May 20 at 10:25
$begingroup$
How about query time $O(log^4 n)$?
$endgroup$
– Yuval Filmus
May 20 at 10:45
$begingroup$
Recently, I read about persistent segment tree, and I think that we can solve this with persistent segment tree, for each prefix of the array we store one segment tree whose leafs actually represent the count of some number $x$. Then to find the sum we actually do binary search on two prefixes of all the roots we save, however I'm not really sure how to finish this and implement since we can have bigger numbers and even duplicates. Careful implementations can run in $O(log N)$
$endgroup$
– someone12321
May 20 at 13:12
add a comment |
$begingroup$
This answer refers to the version of the question in which the range $[l,r]$ refers to indices of the array, and in which we have $Q$ queries. The question asked whether we could beat $O(Qnlog n)$.
Without preprocessing: Using the linear time selection algorithm, you can answer each query in $O(n)$, for a total of $O(Qn)$, which is better than $O(Qnlog n)$.
With preprocessing: Assume for simplicity that $n$ is a power of 2. We consider $log n$ layers, the first consisting of $n$ intervals of length 1, the second of $n/2$ intervals of length 2, and so on: the $k$th consists of $n/2^k$ intervals of length $k$. We sort all of the intervals in $O(nlog n)$ by "merging up the tree". At query time, we partition the input interval into $O(log n)$ stored intervals, and them return the answer in $O(klog n)$ by merging the $k$th largest elements from each.
We can improve the query time as follows. Along with the layered intervals, we also compute their running sums. Let us consider the $O(log n)$ layered intervals $I_1,ldots,I_m$ whose union is the given interval $[l,r]$. The $k$ largest elements of $[l,r]$ are composed of the $k_1,ldots,k_m$ largest elements in $I_1,ldots,I_m$. If we could determine $k_1,ldots,k_m$, then we could compute the answer in $O(m) = O(log n)$ using the running sums.
We can determine $k_1,ldots,k_m$ as follows. For each element $x in I_1$, we can count how many elements are larger than $x$ in time $O(mlog k) = O(log n log k)$ using binary search. By doing binary search on the top $k$ elements of $I_1$, we can determine $k_1$ in time $O(log n log^2 k)$. Continuing in this way, we can determine $k_1,ldots,k_m$ in time $O(log^2 n log^2 k)$.
This solution has preprocessing time $O(nlog n)$ and query time $O(log^2 n log^2 k)$.
We can obtain other tradeoffs by varying the fan-in of the tree. Suppose that the fan-in is $n^{1/d}$. For each internal node in the tree, we sort each interval-of-intervals within its children. At level $i$ we have $n^{i/d}$ vertices, each has $n^{2/d}$ intervals-of-intervals, each of length at most $n^{1-i/d}$, so sorting all intervals-of-intervals at level $i$ takes time $n^{1+2/d}$, for a total preprocessing time of $O(dn^{1+2/d})$. In query time, we can break $[l,r]$ into a union of $O(d)$ intervals, for a total query time of $O(d^2log^2 k)$.
Stated differently, for each $epsilon > 0$ this gives an algorithm with preprocessing time $O(epsilon^{-1} n^{1+epsilon})$ and query time $O(epsilon^{-2} log^2 k)$.
$endgroup$
$begingroup$
I don't think that this improves the things a lot, since k can be up to n.
$endgroup$
– someone12321
May 20 at 10:16
$begingroup$
You can precompute all the answers in $O(n^3)$, and then the time per query is $O(1)$.
$endgroup$
– Yuval Filmus
May 20 at 10:22
$begingroup$
We can even precompute all the answer in $O(n^2 log n)$ however this is very slow and memory consuming solution.
$endgroup$
– someone12321
May 20 at 10:25
$begingroup$
How about query time $O(log^4 n)$?
$endgroup$
– Yuval Filmus
May 20 at 10:45
$begingroup$
Recently, I read about persistent segment tree, and I think that we can solve this with persistent segment tree, for each prefix of the array we store one segment tree whose leafs actually represent the count of some number $x$. Then to find the sum we actually do binary search on two prefixes of all the roots we save, however I'm not really sure how to finish this and implement since we can have bigger numbers and even duplicates. Careful implementations can run in $O(log N)$
$endgroup$
– someone12321
May 20 at 13:12
add a comment |
$begingroup$
This answer refers to the version of the question in which the range $[l,r]$ refers to indices of the array, and in which we have $Q$ queries. The question asked whether we could beat $O(Qnlog n)$.
Without preprocessing: Using the linear time selection algorithm, you can answer each query in $O(n)$, for a total of $O(Qn)$, which is better than $O(Qnlog n)$.
With preprocessing: Assume for simplicity that $n$ is a power of 2. We consider $log n$ layers, the first consisting of $n$ intervals of length 1, the second of $n/2$ intervals of length 2, and so on: the $k$th consists of $n/2^k$ intervals of length $k$. We sort all of the intervals in $O(nlog n)$ by "merging up the tree". At query time, we partition the input interval into $O(log n)$ stored intervals, and them return the answer in $O(klog n)$ by merging the $k$th largest elements from each.
We can improve the query time as follows. Along with the layered intervals, we also compute their running sums. Let us consider the $O(log n)$ layered intervals $I_1,ldots,I_m$ whose union is the given interval $[l,r]$. The $k$ largest elements of $[l,r]$ are composed of the $k_1,ldots,k_m$ largest elements in $I_1,ldots,I_m$. If we could determine $k_1,ldots,k_m$, then we could compute the answer in $O(m) = O(log n)$ using the running sums.
We can determine $k_1,ldots,k_m$ as follows. For each element $x in I_1$, we can count how many elements are larger than $x$ in time $O(mlog k) = O(log n log k)$ using binary search. By doing binary search on the top $k$ elements of $I_1$, we can determine $k_1$ in time $O(log n log^2 k)$. Continuing in this way, we can determine $k_1,ldots,k_m$ in time $O(log^2 n log^2 k)$.
This solution has preprocessing time $O(nlog n)$ and query time $O(log^2 n log^2 k)$.
We can obtain other tradeoffs by varying the fan-in of the tree. Suppose that the fan-in is $n^{1/d}$. For each internal node in the tree, we sort each interval-of-intervals within its children. At level $i$ we have $n^{i/d}$ vertices, each has $n^{2/d}$ intervals-of-intervals, each of length at most $n^{1-i/d}$, so sorting all intervals-of-intervals at level $i$ takes time $n^{1+2/d}$, for a total preprocessing time of $O(dn^{1+2/d})$. In query time, we can break $[l,r]$ into a union of $O(d)$ intervals, for a total query time of $O(d^2log^2 k)$.
Stated differently, for each $epsilon > 0$ this gives an algorithm with preprocessing time $O(epsilon^{-1} n^{1+epsilon})$ and query time $O(epsilon^{-2} log^2 k)$.
$endgroup$
This answer refers to the version of the question in which the range $[l,r]$ refers to indices of the array, and in which we have $Q$ queries. The question asked whether we could beat $O(Qnlog n)$.
Without preprocessing: Using the linear time selection algorithm, you can answer each query in $O(n)$, for a total of $O(Qn)$, which is better than $O(Qnlog n)$.
With preprocessing: Assume for simplicity that $n$ is a power of 2. We consider $log n$ layers, the first consisting of $n$ intervals of length 1, the second of $n/2$ intervals of length 2, and so on: the $k$th consists of $n/2^k$ intervals of length $k$. We sort all of the intervals in $O(nlog n)$ by "merging up the tree". At query time, we partition the input interval into $O(log n)$ stored intervals, and them return the answer in $O(klog n)$ by merging the $k$th largest elements from each.
We can improve the query time as follows. Along with the layered intervals, we also compute their running sums. Let us consider the $O(log n)$ layered intervals $I_1,ldots,I_m$ whose union is the given interval $[l,r]$. The $k$ largest elements of $[l,r]$ are composed of the $k_1,ldots,k_m$ largest elements in $I_1,ldots,I_m$. If we could determine $k_1,ldots,k_m$, then we could compute the answer in $O(m) = O(log n)$ using the running sums.
We can determine $k_1,ldots,k_m$ as follows. For each element $x in I_1$, we can count how many elements are larger than $x$ in time $O(mlog k) = O(log n log k)$ using binary search. By doing binary search on the top $k$ elements of $I_1$, we can determine $k_1$ in time $O(log n log^2 k)$. Continuing in this way, we can determine $k_1,ldots,k_m$ in time $O(log^2 n log^2 k)$.
This solution has preprocessing time $O(nlog n)$ and query time $O(log^2 n log^2 k)$.
We can obtain other tradeoffs by varying the fan-in of the tree. Suppose that the fan-in is $n^{1/d}$. For each internal node in the tree, we sort each interval-of-intervals within its children. At level $i$ we have $n^{i/d}$ vertices, each has $n^{2/d}$ intervals-of-intervals, each of length at most $n^{1-i/d}$, so sorting all intervals-of-intervals at level $i$ takes time $n^{1+2/d}$, for a total preprocessing time of $O(dn^{1+2/d})$. In query time, we can break $[l,r]$ into a union of $O(d)$ intervals, for a total query time of $O(d^2log^2 k)$.
Stated differently, for each $epsilon > 0$ this gives an algorithm with preprocessing time $O(epsilon^{-1} n^{1+epsilon})$ and query time $O(epsilon^{-2} log^2 k)$.
edited May 20 at 10:57
answered May 20 at 9:53


Yuval FilmusYuval Filmus
205k15 gold badges200 silver badges365 bronze badges
205k15 gold badges200 silver badges365 bronze badges
$begingroup$
I don't think that this improves the things a lot, since k can be up to n.
$endgroup$
– someone12321
May 20 at 10:16
$begingroup$
You can precompute all the answers in $O(n^3)$, and then the time per query is $O(1)$.
$endgroup$
– Yuval Filmus
May 20 at 10:22
$begingroup$
We can even precompute all the answer in $O(n^2 log n)$ however this is very slow and memory consuming solution.
$endgroup$
– someone12321
May 20 at 10:25
$begingroup$
How about query time $O(log^4 n)$?
$endgroup$
– Yuval Filmus
May 20 at 10:45
$begingroup$
Recently, I read about persistent segment tree, and I think that we can solve this with persistent segment tree, for each prefix of the array we store one segment tree whose leafs actually represent the count of some number $x$. Then to find the sum we actually do binary search on two prefixes of all the roots we save, however I'm not really sure how to finish this and implement since we can have bigger numbers and even duplicates. Careful implementations can run in $O(log N)$
$endgroup$
– someone12321
May 20 at 13:12
add a comment |
$begingroup$
I don't think that this improves the things a lot, since k can be up to n.
$endgroup$
– someone12321
May 20 at 10:16
$begingroup$
You can precompute all the answers in $O(n^3)$, and then the time per query is $O(1)$.
$endgroup$
– Yuval Filmus
May 20 at 10:22
$begingroup$
We can even precompute all the answer in $O(n^2 log n)$ however this is very slow and memory consuming solution.
$endgroup$
– someone12321
May 20 at 10:25
$begingroup$
How about query time $O(log^4 n)$?
$endgroup$
– Yuval Filmus
May 20 at 10:45
$begingroup$
Recently, I read about persistent segment tree, and I think that we can solve this with persistent segment tree, for each prefix of the array we store one segment tree whose leafs actually represent the count of some number $x$. Then to find the sum we actually do binary search on two prefixes of all the roots we save, however I'm not really sure how to finish this and implement since we can have bigger numbers and even duplicates. Careful implementations can run in $O(log N)$
$endgroup$
– someone12321
May 20 at 13:12
$begingroup$
I don't think that this improves the things a lot, since k can be up to n.
$endgroup$
– someone12321
May 20 at 10:16
$begingroup$
I don't think that this improves the things a lot, since k can be up to n.
$endgroup$
– someone12321
May 20 at 10:16
$begingroup$
You can precompute all the answers in $O(n^3)$, and then the time per query is $O(1)$.
$endgroup$
– Yuval Filmus
May 20 at 10:22
$begingroup$
You can precompute all the answers in $O(n^3)$, and then the time per query is $O(1)$.
$endgroup$
– Yuval Filmus
May 20 at 10:22
$begingroup$
We can even precompute all the answer in $O(n^2 log n)$ however this is very slow and memory consuming solution.
$endgroup$
– someone12321
May 20 at 10:25
$begingroup$
We can even precompute all the answer in $O(n^2 log n)$ however this is very slow and memory consuming solution.
$endgroup$
– someone12321
May 20 at 10:25
$begingroup$
How about query time $O(log^4 n)$?
$endgroup$
– Yuval Filmus
May 20 at 10:45
$begingroup$
How about query time $O(log^4 n)$?
$endgroup$
– Yuval Filmus
May 20 at 10:45
$begingroup$
Recently, I read about persistent segment tree, and I think that we can solve this with persistent segment tree, for each prefix of the array we store one segment tree whose leafs actually represent the count of some number $x$. Then to find the sum we actually do binary search on two prefixes of all the roots we save, however I'm not really sure how to finish this and implement since we can have bigger numbers and even duplicates. Careful implementations can run in $O(log N)$
$endgroup$
– someone12321
May 20 at 13:12
$begingroup$
Recently, I read about persistent segment tree, and I think that we can solve this with persistent segment tree, for each prefix of the array we store one segment tree whose leafs actually represent the count of some number $x$. Then to find the sum we actually do binary search on two prefixes of all the roots we save, however I'm not really sure how to finish this and implement since we can have bigger numbers and even duplicates. Careful implementations can run in $O(log N)$
$endgroup$
– someone12321
May 20 at 13:12
add a comment |
Thanks for contributing an answer to Computer Science Stack Exchange!
- Please be sure to answer the question. Provide details and share your research!
But avoid …
- Asking for help, clarification, or responding to other answers.
- Making statements based on opinion; back them up with references or personal experience.
Use MathJax to format equations. MathJax reference.
To learn more, see our tips on writing great answers.
Sign up or log in
StackExchange.ready(function () {
StackExchange.helpers.onClickDraftSave('#login-link');
});
Sign up using Google
Sign up using Facebook
Sign up using Email and Password
Post as a guest
Required, but never shown
StackExchange.ready(
function () {
StackExchange.openid.initPostLogin('.new-post-login', 'https%3a%2f%2fcs.stackexchange.com%2fquestions%2f109579%2fhow-to-find-sum-of-maximum-k-elements-in-range-in-array%23new-answer', 'question_page');
}
);
Post as a guest
Required, but never shown
Sign up or log in
StackExchange.ready(function () {
StackExchange.helpers.onClickDraftSave('#login-link');
});
Sign up using Google
Sign up using Facebook
Sign up using Email and Password
Post as a guest
Required, but never shown
Sign up or log in
StackExchange.ready(function () {
StackExchange.helpers.onClickDraftSave('#login-link');
});
Sign up using Google
Sign up using Facebook
Sign up using Email and Password
Post as a guest
Required, but never shown
Sign up or log in
StackExchange.ready(function () {
StackExchange.helpers.onClickDraftSave('#login-link');
});
Sign up using Google
Sign up using Facebook
Sign up using Email and Password
Sign up using Google
Sign up using Facebook
Sign up using Email and Password
Post as a guest
Required, but never shown
Required, but never shown
Required, but never shown
Required, but never shown
Required, but never shown
Required, but never shown
Required, but never shown
Required, but never shown
Required, but never shown
Zrtr3HitoiEx7UJwbKz2JO,0OoNBSimiUgX
$begingroup$
There is eventually a trivial $O(Nk)$.
$endgroup$
– Vince
May 20 at 8:07
$begingroup$
Taking $Q = 1$, you are effectively not allowing any preprocessing at all.
$endgroup$
– Yuval Filmus
May 20 at 9:52