What is the reasoning behind standardization (dividing by standard deviation)?Doubts about the standardization process that I should perform with a set of variablesWhat is a good paper or book to understand standardization and normalization of data with different units of measurement?Centering and scaling skewed distributionsz-score normalisation - how to achieve this for score combination?centering and scaling (standardizing) a variable: use population or sample standard deviation?Standardization of the predictors in regressionWhen I normalize the standardized values of data, why do I get the same values as just normalizing?“We have to apply feature scaling on test set using scaling parameters from train set.” Is this statement true? If yes, why?Normalization vs StandadizationWhat are the different influences of outliers regarding the feature scaling methods: standardization VS. normalization?
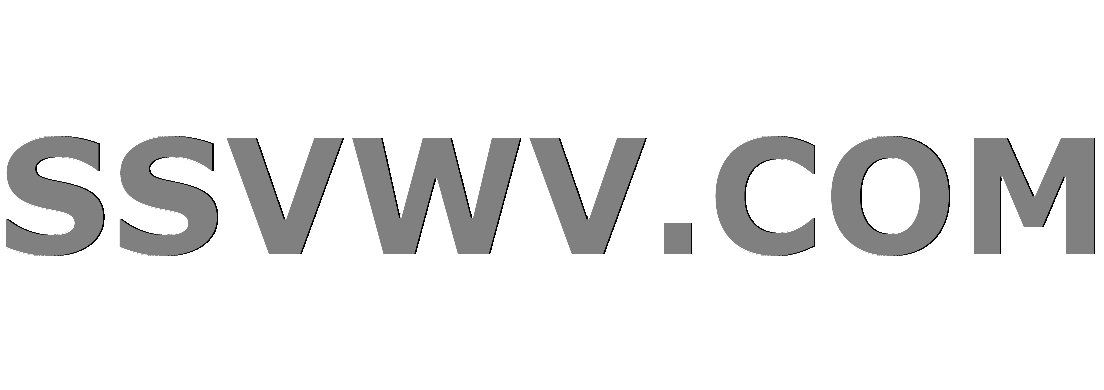
Multi tool use
Language involving irrational number is not a CFL
Logistic function with a slope but no asymptotes?
What is this high flying aircraft over Pennsylvania?
Given this phrasing in the lease, when should I pay my rent?
Does the Crossbow Expert feat's extra crossbow attack work with the reaction attack from a Hunter ranger's Giant Killer feature?
Can I cause damage to electrical appliances by unplugging them when they are turned on?
What the heck is gets(stdin) on site coderbyte?
How do I fix the group tension caused by my character stealing and possibly killing without provocation?
Why didn't Voldemort know what Grindelwald looked like?
Unable to disable Microsoft Store in domain environment
Review your own paper in Mathematics
Why do Radio Buttons not fill the entire outer circle?
In One Punch Man, is King actually weak?
Why is participating in the European Parliamentary elections used as a threat?
What does "tick" mean in this sentence?
Why didn’t Eve recognize the little cockroach as a living organism?
Limit max CPU usage SQL SERVER with WSRM
Using streams for a null-safe conversion from an array to list
How can I, as DM, avoid the Conga Line of Death occurring when implementing some form of flanking rule?
Typing CO_2 easily
What is the meaning of the following sentence?
Are Captain Marvel's powers affected by Thanos breaking the Tesseract and claiming the stone?
Do you waste sorcery points if you try to apply metamagic to a spell from a scroll but fail to cast it?
Why Shazam when there is already Superman?
What is the reasoning behind standardization (dividing by standard deviation)?
Doubts about the standardization process that I should perform with a set of variablesWhat is a good paper or book to understand standardization and normalization of data with different units of measurement?Centering and scaling skewed distributionsz-score normalisation - how to achieve this for score combination?centering and scaling (standardizing) a variable: use population or sample standard deviation?Standardization of the predictors in regressionWhen I normalize the standardized values of data, why do I get the same values as just normalizing?“We have to apply feature scaling on test set using scaling parameters from train set.” Is this statement true? If yes, why?Normalization vs StandadizationWhat are the different influences of outliers regarding the feature scaling methods: standardization VS. normalization?
$begingroup$
Why does dividing a dataset by sigma make the sample variance equal to 1? Assuming a zero mean for simplicity.
What's the intuition behind this?
Dividing by the range (max-min) makes intuitive sense. But standard deviation does not.
standardization
$endgroup$
add a comment |
$begingroup$
Why does dividing a dataset by sigma make the sample variance equal to 1? Assuming a zero mean for simplicity.
What's the intuition behind this?
Dividing by the range (max-min) makes intuitive sense. But standard deviation does not.
standardization
$endgroup$
1
$begingroup$
The zero mean assumption isn't necessary. You can take this as three separate statements: dividing by SD gives an SD of 1; the variance is the square of the SD; and the square of 1 is 1.
$endgroup$
– Nick Cox
2 days ago
1
$begingroup$
When people say intuitive, I translate that as "familiar to me", and most of the time it fits. Reasons for not dividing by the range are practical rather than theoretical. The range can be highly labile. Also, often the range of all values is enormously larger than the that of the bulk of the values, so the results wouldn't be very helpful. Income illustrates both points: the observed maximum may vary capriciously and values divided by the range would often be concentrated near 0.
$endgroup$
– Nick Cox
2 days ago
add a comment |
$begingroup$
Why does dividing a dataset by sigma make the sample variance equal to 1? Assuming a zero mean for simplicity.
What's the intuition behind this?
Dividing by the range (max-min) makes intuitive sense. But standard deviation does not.
standardization
$endgroup$
Why does dividing a dataset by sigma make the sample variance equal to 1? Assuming a zero mean for simplicity.
What's the intuition behind this?
Dividing by the range (max-min) makes intuitive sense. But standard deviation does not.
standardization
standardization
edited 2 days ago
Karolis Koncevičius
2,09941527
2,09941527
asked 2 days ago


alwayscuriousalwayscurious
1985
1985
1
$begingroup$
The zero mean assumption isn't necessary. You can take this as three separate statements: dividing by SD gives an SD of 1; the variance is the square of the SD; and the square of 1 is 1.
$endgroup$
– Nick Cox
2 days ago
1
$begingroup$
When people say intuitive, I translate that as "familiar to me", and most of the time it fits. Reasons for not dividing by the range are practical rather than theoretical. The range can be highly labile. Also, often the range of all values is enormously larger than the that of the bulk of the values, so the results wouldn't be very helpful. Income illustrates both points: the observed maximum may vary capriciously and values divided by the range would often be concentrated near 0.
$endgroup$
– Nick Cox
2 days ago
add a comment |
1
$begingroup$
The zero mean assumption isn't necessary. You can take this as three separate statements: dividing by SD gives an SD of 1; the variance is the square of the SD; and the square of 1 is 1.
$endgroup$
– Nick Cox
2 days ago
1
$begingroup$
When people say intuitive, I translate that as "familiar to me", and most of the time it fits. Reasons for not dividing by the range are practical rather than theoretical. The range can be highly labile. Also, often the range of all values is enormously larger than the that of the bulk of the values, so the results wouldn't be very helpful. Income illustrates both points: the observed maximum may vary capriciously and values divided by the range would often be concentrated near 0.
$endgroup$
– Nick Cox
2 days ago
1
1
$begingroup$
The zero mean assumption isn't necessary. You can take this as three separate statements: dividing by SD gives an SD of 1; the variance is the square of the SD; and the square of 1 is 1.
$endgroup$
– Nick Cox
2 days ago
$begingroup$
The zero mean assumption isn't necessary. You can take this as three separate statements: dividing by SD gives an SD of 1; the variance is the square of the SD; and the square of 1 is 1.
$endgroup$
– Nick Cox
2 days ago
1
1
$begingroup$
When people say intuitive, I translate that as "familiar to me", and most of the time it fits. Reasons for not dividing by the range are practical rather than theoretical. The range can be highly labile. Also, often the range of all values is enormously larger than the that of the bulk of the values, so the results wouldn't be very helpful. Income illustrates both points: the observed maximum may vary capriciously and values divided by the range would often be concentrated near 0.
$endgroup$
– Nick Cox
2 days ago
$begingroup$
When people say intuitive, I translate that as "familiar to me", and most of the time it fits. Reasons for not dividing by the range are practical rather than theoretical. The range can be highly labile. Also, often the range of all values is enormously larger than the that of the bulk of the values, so the results wouldn't be very helpful. Income illustrates both points: the observed maximum may vary capriciously and values divided by the range would often be concentrated near 0.
$endgroup$
– Nick Cox
2 days ago
add a comment |
2 Answers
2
active
oldest
votes
$begingroup$
This stems from the property of variance. For a random variable $X$ and a constant $a$, $mathrmvar(aX)=a^2mathrmvar(x)$. Therefore, if you divide the data by its standard deviation ($sigma$), $mathrmvar(X/sigma)=mathrmvar(X)/sigma^2=sigma^2/sigma^2=1$.
New contributor
Chao Song is a new contributor to this site. Take care in asking for clarification, commenting, and answering.
Check out our Code of Conduct.
$endgroup$
1
$begingroup$
that helps, thanks. Do you have an intuitive approach?
$endgroup$
– alwayscurious
2 days ago
add a comment |
$begingroup$
Standardizing is is just changing the units so they are in "standard deviation" units. After standardization, a value of 1.5 means "1.5 standard deviations above 0". If the standard deviation were 8, this would be equivalent to saying "12 points above 0".
An example: when converting inches to feet (in America), you multiply your data in inches by a conversion factor, $frac1 foot12 inches$, which comes from the fact that 1 foot equals 12 inches, so you're essentially just multiplying your data points by a fancy version of 1 (i.e., a fraction with equal numerator and denominator). For example, to go from 72 inches to feet, you do $72 inches times frac1 foot12 inches=6feet$.
When converting scores from raw units to standard deviation units, you multiply your data in raw units by the conversion factor $frac1sdsigma points$. So if you had a score of 100 and the standard deviation ($sigma$) was 20, your standardized score would be $100 points times frac1 sd20 points=5sd$. Standardization is just changing the units.
Changing the units of a dataset doesn't affect how spread out it is; you just change the units of the measure of spread you're using so that they match. So if your original data had a standard deviation of 20 points, and you've changed units so that 20 original points equals 1 new standardized unit, then the new standard deviation is 1 unit (because 20 original units equals 1 new unit).
$endgroup$
2
$begingroup$
Some of your answer needs an extra assumption that you have subtracted the mean, but you don't mention that. The thread question is equivocal here too, as in statistics subtracting the mean is the default, but it asks only about dividing by the SD.
$endgroup$
– Nick Cox
2 days ago
$begingroup$
I don't think my answer requires that assumption if we're defining standardization as just dividing by the SD (which OP does). I'm just talking about a change of units, not with reference to the center of the data. E.g., for a scale with a mean of 50 and an SD of 10, I'm saying a score of 20 would have a standardized score of 2, not -3. Subtracting the mean (centering) is a separate issue.
$endgroup$
– Noah
yesterday
$begingroup$
Fair point. I don't think defining standardization as merely dividing by the SD is at all standard, so to speak, but granting your definition that value / SD $=: z$, say, then all data points that are positive are then above 0 on the standardized $z$ scale and only points that happen to be negative are below 0 on the $z$ scale. Whether that is as useful a standardization as (value $-$ mean) / SD is open to question.
$endgroup$
– Nick Cox
yesterday
add a comment |
Your Answer
StackExchange.ifUsing("editor", function ()
return StackExchange.using("mathjaxEditing", function ()
StackExchange.MarkdownEditor.creationCallbacks.add(function (editor, postfix)
StackExchange.mathjaxEditing.prepareWmdForMathJax(editor, postfix, [["$", "$"], ["\\(","\\)"]]);
);
);
, "mathjax-editing");
StackExchange.ready(function()
var channelOptions =
tags: "".split(" "),
id: "65"
;
initTagRenderer("".split(" "), "".split(" "), channelOptions);
StackExchange.using("externalEditor", function()
// Have to fire editor after snippets, if snippets enabled
if (StackExchange.settings.snippets.snippetsEnabled)
StackExchange.using("snippets", function()
createEditor();
);
else
createEditor();
);
function createEditor()
StackExchange.prepareEditor(
heartbeatType: 'answer',
autoActivateHeartbeat: false,
convertImagesToLinks: false,
noModals: true,
showLowRepImageUploadWarning: true,
reputationToPostImages: null,
bindNavPrevention: true,
postfix: "",
imageUploader:
brandingHtml: "Powered by u003ca class="icon-imgur-white" href="https://imgur.com/"u003eu003c/au003e",
contentPolicyHtml: "User contributions licensed under u003ca href="https://creativecommons.org/licenses/by-sa/3.0/"u003ecc by-sa 3.0 with attribution requiredu003c/au003e u003ca href="https://stackoverflow.com/legal/content-policy"u003e(content policy)u003c/au003e",
allowUrls: true
,
onDemand: true,
discardSelector: ".discard-answer"
,immediatelyShowMarkdownHelp:true
);
);
Sign up or log in
StackExchange.ready(function ()
StackExchange.helpers.onClickDraftSave('#login-link');
);
Sign up using Google
Sign up using Facebook
Sign up using Email and Password
Post as a guest
Required, but never shown
StackExchange.ready(
function ()
StackExchange.openid.initPostLogin('.new-post-login', 'https%3a%2f%2fstats.stackexchange.com%2fquestions%2f398116%2fwhat-is-the-reasoning-behind-standardization-dividing-by-standard-deviation%23new-answer', 'question_page');
);
Post as a guest
Required, but never shown
2 Answers
2
active
oldest
votes
2 Answers
2
active
oldest
votes
active
oldest
votes
active
oldest
votes
$begingroup$
This stems from the property of variance. For a random variable $X$ and a constant $a$, $mathrmvar(aX)=a^2mathrmvar(x)$. Therefore, if you divide the data by its standard deviation ($sigma$), $mathrmvar(X/sigma)=mathrmvar(X)/sigma^2=sigma^2/sigma^2=1$.
New contributor
Chao Song is a new contributor to this site. Take care in asking for clarification, commenting, and answering.
Check out our Code of Conduct.
$endgroup$
1
$begingroup$
that helps, thanks. Do you have an intuitive approach?
$endgroup$
– alwayscurious
2 days ago
add a comment |
$begingroup$
This stems from the property of variance. For a random variable $X$ and a constant $a$, $mathrmvar(aX)=a^2mathrmvar(x)$. Therefore, if you divide the data by its standard deviation ($sigma$), $mathrmvar(X/sigma)=mathrmvar(X)/sigma^2=sigma^2/sigma^2=1$.
New contributor
Chao Song is a new contributor to this site. Take care in asking for clarification, commenting, and answering.
Check out our Code of Conduct.
$endgroup$
1
$begingroup$
that helps, thanks. Do you have an intuitive approach?
$endgroup$
– alwayscurious
2 days ago
add a comment |
$begingroup$
This stems from the property of variance. For a random variable $X$ and a constant $a$, $mathrmvar(aX)=a^2mathrmvar(x)$. Therefore, if you divide the data by its standard deviation ($sigma$), $mathrmvar(X/sigma)=mathrmvar(X)/sigma^2=sigma^2/sigma^2=1$.
New contributor
Chao Song is a new contributor to this site. Take care in asking for clarification, commenting, and answering.
Check out our Code of Conduct.
$endgroup$
This stems from the property of variance. For a random variable $X$ and a constant $a$, $mathrmvar(aX)=a^2mathrmvar(x)$. Therefore, if you divide the data by its standard deviation ($sigma$), $mathrmvar(X/sigma)=mathrmvar(X)/sigma^2=sigma^2/sigma^2=1$.
New contributor
Chao Song is a new contributor to this site. Take care in asking for clarification, commenting, and answering.
Check out our Code of Conduct.
New contributor
Chao Song is a new contributor to this site. Take care in asking for clarification, commenting, and answering.
Check out our Code of Conduct.
answered 2 days ago
Chao SongChao Song
2015
2015
New contributor
Chao Song is a new contributor to this site. Take care in asking for clarification, commenting, and answering.
Check out our Code of Conduct.
New contributor
Chao Song is a new contributor to this site. Take care in asking for clarification, commenting, and answering.
Check out our Code of Conduct.
Chao Song is a new contributor to this site. Take care in asking for clarification, commenting, and answering.
Check out our Code of Conduct.
1
$begingroup$
that helps, thanks. Do you have an intuitive approach?
$endgroup$
– alwayscurious
2 days ago
add a comment |
1
$begingroup$
that helps, thanks. Do you have an intuitive approach?
$endgroup$
– alwayscurious
2 days ago
1
1
$begingroup$
that helps, thanks. Do you have an intuitive approach?
$endgroup$
– alwayscurious
2 days ago
$begingroup$
that helps, thanks. Do you have an intuitive approach?
$endgroup$
– alwayscurious
2 days ago
add a comment |
$begingroup$
Standardizing is is just changing the units so they are in "standard deviation" units. After standardization, a value of 1.5 means "1.5 standard deviations above 0". If the standard deviation were 8, this would be equivalent to saying "12 points above 0".
An example: when converting inches to feet (in America), you multiply your data in inches by a conversion factor, $frac1 foot12 inches$, which comes from the fact that 1 foot equals 12 inches, so you're essentially just multiplying your data points by a fancy version of 1 (i.e., a fraction with equal numerator and denominator). For example, to go from 72 inches to feet, you do $72 inches times frac1 foot12 inches=6feet$.
When converting scores from raw units to standard deviation units, you multiply your data in raw units by the conversion factor $frac1sdsigma points$. So if you had a score of 100 and the standard deviation ($sigma$) was 20, your standardized score would be $100 points times frac1 sd20 points=5sd$. Standardization is just changing the units.
Changing the units of a dataset doesn't affect how spread out it is; you just change the units of the measure of spread you're using so that they match. So if your original data had a standard deviation of 20 points, and you've changed units so that 20 original points equals 1 new standardized unit, then the new standard deviation is 1 unit (because 20 original units equals 1 new unit).
$endgroup$
2
$begingroup$
Some of your answer needs an extra assumption that you have subtracted the mean, but you don't mention that. The thread question is equivocal here too, as in statistics subtracting the mean is the default, but it asks only about dividing by the SD.
$endgroup$
– Nick Cox
2 days ago
$begingroup$
I don't think my answer requires that assumption if we're defining standardization as just dividing by the SD (which OP does). I'm just talking about a change of units, not with reference to the center of the data. E.g., for a scale with a mean of 50 and an SD of 10, I'm saying a score of 20 would have a standardized score of 2, not -3. Subtracting the mean (centering) is a separate issue.
$endgroup$
– Noah
yesterday
$begingroup$
Fair point. I don't think defining standardization as merely dividing by the SD is at all standard, so to speak, but granting your definition that value / SD $=: z$, say, then all data points that are positive are then above 0 on the standardized $z$ scale and only points that happen to be negative are below 0 on the $z$ scale. Whether that is as useful a standardization as (value $-$ mean) / SD is open to question.
$endgroup$
– Nick Cox
yesterday
add a comment |
$begingroup$
Standardizing is is just changing the units so they are in "standard deviation" units. After standardization, a value of 1.5 means "1.5 standard deviations above 0". If the standard deviation were 8, this would be equivalent to saying "12 points above 0".
An example: when converting inches to feet (in America), you multiply your data in inches by a conversion factor, $frac1 foot12 inches$, which comes from the fact that 1 foot equals 12 inches, so you're essentially just multiplying your data points by a fancy version of 1 (i.e., a fraction with equal numerator and denominator). For example, to go from 72 inches to feet, you do $72 inches times frac1 foot12 inches=6feet$.
When converting scores from raw units to standard deviation units, you multiply your data in raw units by the conversion factor $frac1sdsigma points$. So if you had a score of 100 and the standard deviation ($sigma$) was 20, your standardized score would be $100 points times frac1 sd20 points=5sd$. Standardization is just changing the units.
Changing the units of a dataset doesn't affect how spread out it is; you just change the units of the measure of spread you're using so that they match. So if your original data had a standard deviation of 20 points, and you've changed units so that 20 original points equals 1 new standardized unit, then the new standard deviation is 1 unit (because 20 original units equals 1 new unit).
$endgroup$
2
$begingroup$
Some of your answer needs an extra assumption that you have subtracted the mean, but you don't mention that. The thread question is equivocal here too, as in statistics subtracting the mean is the default, but it asks only about dividing by the SD.
$endgroup$
– Nick Cox
2 days ago
$begingroup$
I don't think my answer requires that assumption if we're defining standardization as just dividing by the SD (which OP does). I'm just talking about a change of units, not with reference to the center of the data. E.g., for a scale with a mean of 50 and an SD of 10, I'm saying a score of 20 would have a standardized score of 2, not -3. Subtracting the mean (centering) is a separate issue.
$endgroup$
– Noah
yesterday
$begingroup$
Fair point. I don't think defining standardization as merely dividing by the SD is at all standard, so to speak, but granting your definition that value / SD $=: z$, say, then all data points that are positive are then above 0 on the standardized $z$ scale and only points that happen to be negative are below 0 on the $z$ scale. Whether that is as useful a standardization as (value $-$ mean) / SD is open to question.
$endgroup$
– Nick Cox
yesterday
add a comment |
$begingroup$
Standardizing is is just changing the units so they are in "standard deviation" units. After standardization, a value of 1.5 means "1.5 standard deviations above 0". If the standard deviation were 8, this would be equivalent to saying "12 points above 0".
An example: when converting inches to feet (in America), you multiply your data in inches by a conversion factor, $frac1 foot12 inches$, which comes from the fact that 1 foot equals 12 inches, so you're essentially just multiplying your data points by a fancy version of 1 (i.e., a fraction with equal numerator and denominator). For example, to go from 72 inches to feet, you do $72 inches times frac1 foot12 inches=6feet$.
When converting scores from raw units to standard deviation units, you multiply your data in raw units by the conversion factor $frac1sdsigma points$. So if you had a score of 100 and the standard deviation ($sigma$) was 20, your standardized score would be $100 points times frac1 sd20 points=5sd$. Standardization is just changing the units.
Changing the units of a dataset doesn't affect how spread out it is; you just change the units of the measure of spread you're using so that they match. So if your original data had a standard deviation of 20 points, and you've changed units so that 20 original points equals 1 new standardized unit, then the new standard deviation is 1 unit (because 20 original units equals 1 new unit).
$endgroup$
Standardizing is is just changing the units so they are in "standard deviation" units. After standardization, a value of 1.5 means "1.5 standard deviations above 0". If the standard deviation were 8, this would be equivalent to saying "12 points above 0".
An example: when converting inches to feet (in America), you multiply your data in inches by a conversion factor, $frac1 foot12 inches$, which comes from the fact that 1 foot equals 12 inches, so you're essentially just multiplying your data points by a fancy version of 1 (i.e., a fraction with equal numerator and denominator). For example, to go from 72 inches to feet, you do $72 inches times frac1 foot12 inches=6feet$.
When converting scores from raw units to standard deviation units, you multiply your data in raw units by the conversion factor $frac1sdsigma points$. So if you had a score of 100 and the standard deviation ($sigma$) was 20, your standardized score would be $100 points times frac1 sd20 points=5sd$. Standardization is just changing the units.
Changing the units of a dataset doesn't affect how spread out it is; you just change the units of the measure of spread you're using so that they match. So if your original data had a standard deviation of 20 points, and you've changed units so that 20 original points equals 1 new standardized unit, then the new standard deviation is 1 unit (because 20 original units equals 1 new unit).
edited yesterday
answered 2 days ago
NoahNoah
3,3161316
3,3161316
2
$begingroup$
Some of your answer needs an extra assumption that you have subtracted the mean, but you don't mention that. The thread question is equivocal here too, as in statistics subtracting the mean is the default, but it asks only about dividing by the SD.
$endgroup$
– Nick Cox
2 days ago
$begingroup$
I don't think my answer requires that assumption if we're defining standardization as just dividing by the SD (which OP does). I'm just talking about a change of units, not with reference to the center of the data. E.g., for a scale with a mean of 50 and an SD of 10, I'm saying a score of 20 would have a standardized score of 2, not -3. Subtracting the mean (centering) is a separate issue.
$endgroup$
– Noah
yesterday
$begingroup$
Fair point. I don't think defining standardization as merely dividing by the SD is at all standard, so to speak, but granting your definition that value / SD $=: z$, say, then all data points that are positive are then above 0 on the standardized $z$ scale and only points that happen to be negative are below 0 on the $z$ scale. Whether that is as useful a standardization as (value $-$ mean) / SD is open to question.
$endgroup$
– Nick Cox
yesterday
add a comment |
2
$begingroup$
Some of your answer needs an extra assumption that you have subtracted the mean, but you don't mention that. The thread question is equivocal here too, as in statistics subtracting the mean is the default, but it asks only about dividing by the SD.
$endgroup$
– Nick Cox
2 days ago
$begingroup$
I don't think my answer requires that assumption if we're defining standardization as just dividing by the SD (which OP does). I'm just talking about a change of units, not with reference to the center of the data. E.g., for a scale with a mean of 50 and an SD of 10, I'm saying a score of 20 would have a standardized score of 2, not -3. Subtracting the mean (centering) is a separate issue.
$endgroup$
– Noah
yesterday
$begingroup$
Fair point. I don't think defining standardization as merely dividing by the SD is at all standard, so to speak, but granting your definition that value / SD $=: z$, say, then all data points that are positive are then above 0 on the standardized $z$ scale and only points that happen to be negative are below 0 on the $z$ scale. Whether that is as useful a standardization as (value $-$ mean) / SD is open to question.
$endgroup$
– Nick Cox
yesterday
2
2
$begingroup$
Some of your answer needs an extra assumption that you have subtracted the mean, but you don't mention that. The thread question is equivocal here too, as in statistics subtracting the mean is the default, but it asks only about dividing by the SD.
$endgroup$
– Nick Cox
2 days ago
$begingroup$
Some of your answer needs an extra assumption that you have subtracted the mean, but you don't mention that. The thread question is equivocal here too, as in statistics subtracting the mean is the default, but it asks only about dividing by the SD.
$endgroup$
– Nick Cox
2 days ago
$begingroup$
I don't think my answer requires that assumption if we're defining standardization as just dividing by the SD (which OP does). I'm just talking about a change of units, not with reference to the center of the data. E.g., for a scale with a mean of 50 and an SD of 10, I'm saying a score of 20 would have a standardized score of 2, not -3. Subtracting the mean (centering) is a separate issue.
$endgroup$
– Noah
yesterday
$begingroup$
I don't think my answer requires that assumption if we're defining standardization as just dividing by the SD (which OP does). I'm just talking about a change of units, not with reference to the center of the data. E.g., for a scale with a mean of 50 and an SD of 10, I'm saying a score of 20 would have a standardized score of 2, not -3. Subtracting the mean (centering) is a separate issue.
$endgroup$
– Noah
yesterday
$begingroup$
Fair point. I don't think defining standardization as merely dividing by the SD is at all standard, so to speak, but granting your definition that value / SD $=: z$, say, then all data points that are positive are then above 0 on the standardized $z$ scale and only points that happen to be negative are below 0 on the $z$ scale. Whether that is as useful a standardization as (value $-$ mean) / SD is open to question.
$endgroup$
– Nick Cox
yesterday
$begingroup$
Fair point. I don't think defining standardization as merely dividing by the SD is at all standard, so to speak, but granting your definition that value / SD $=: z$, say, then all data points that are positive are then above 0 on the standardized $z$ scale and only points that happen to be negative are below 0 on the $z$ scale. Whether that is as useful a standardization as (value $-$ mean) / SD is open to question.
$endgroup$
– Nick Cox
yesterday
add a comment |
Thanks for contributing an answer to Cross Validated!
- Please be sure to answer the question. Provide details and share your research!
But avoid …
- Asking for help, clarification, or responding to other answers.
- Making statements based on opinion; back them up with references or personal experience.
Use MathJax to format equations. MathJax reference.
To learn more, see our tips on writing great answers.
Sign up or log in
StackExchange.ready(function ()
StackExchange.helpers.onClickDraftSave('#login-link');
);
Sign up using Google
Sign up using Facebook
Sign up using Email and Password
Post as a guest
Required, but never shown
StackExchange.ready(
function ()
StackExchange.openid.initPostLogin('.new-post-login', 'https%3a%2f%2fstats.stackexchange.com%2fquestions%2f398116%2fwhat-is-the-reasoning-behind-standardization-dividing-by-standard-deviation%23new-answer', 'question_page');
);
Post as a guest
Required, but never shown
Sign up or log in
StackExchange.ready(function ()
StackExchange.helpers.onClickDraftSave('#login-link');
);
Sign up using Google
Sign up using Facebook
Sign up using Email and Password
Post as a guest
Required, but never shown
Sign up or log in
StackExchange.ready(function ()
StackExchange.helpers.onClickDraftSave('#login-link');
);
Sign up using Google
Sign up using Facebook
Sign up using Email and Password
Post as a guest
Required, but never shown
Sign up or log in
StackExchange.ready(function ()
StackExchange.helpers.onClickDraftSave('#login-link');
);
Sign up using Google
Sign up using Facebook
Sign up using Email and Password
Sign up using Google
Sign up using Facebook
Sign up using Email and Password
Post as a guest
Required, but never shown
Required, but never shown
Required, but never shown
Required, but never shown
Required, but never shown
Required, but never shown
Required, but never shown
Required, but never shown
Required, but never shown
ug q,fIc fy zNGMYrQ8jphRq2XukdADl6Ql9AmeGC1N znGpSB3imTj9zpm4swP5kFRh 2X5kM2 r88ZHzG
1
$begingroup$
The zero mean assumption isn't necessary. You can take this as three separate statements: dividing by SD gives an SD of 1; the variance is the square of the SD; and the square of 1 is 1.
$endgroup$
– Nick Cox
2 days ago
1
$begingroup$
When people say intuitive, I translate that as "familiar to me", and most of the time it fits. Reasons for not dividing by the range are practical rather than theoretical. The range can be highly labile. Also, often the range of all values is enormously larger than the that of the bulk of the values, so the results wouldn't be very helpful. Income illustrates both points: the observed maximum may vary capriciously and values divided by the range would often be concentrated near 0.
$endgroup$
– Nick Cox
2 days ago